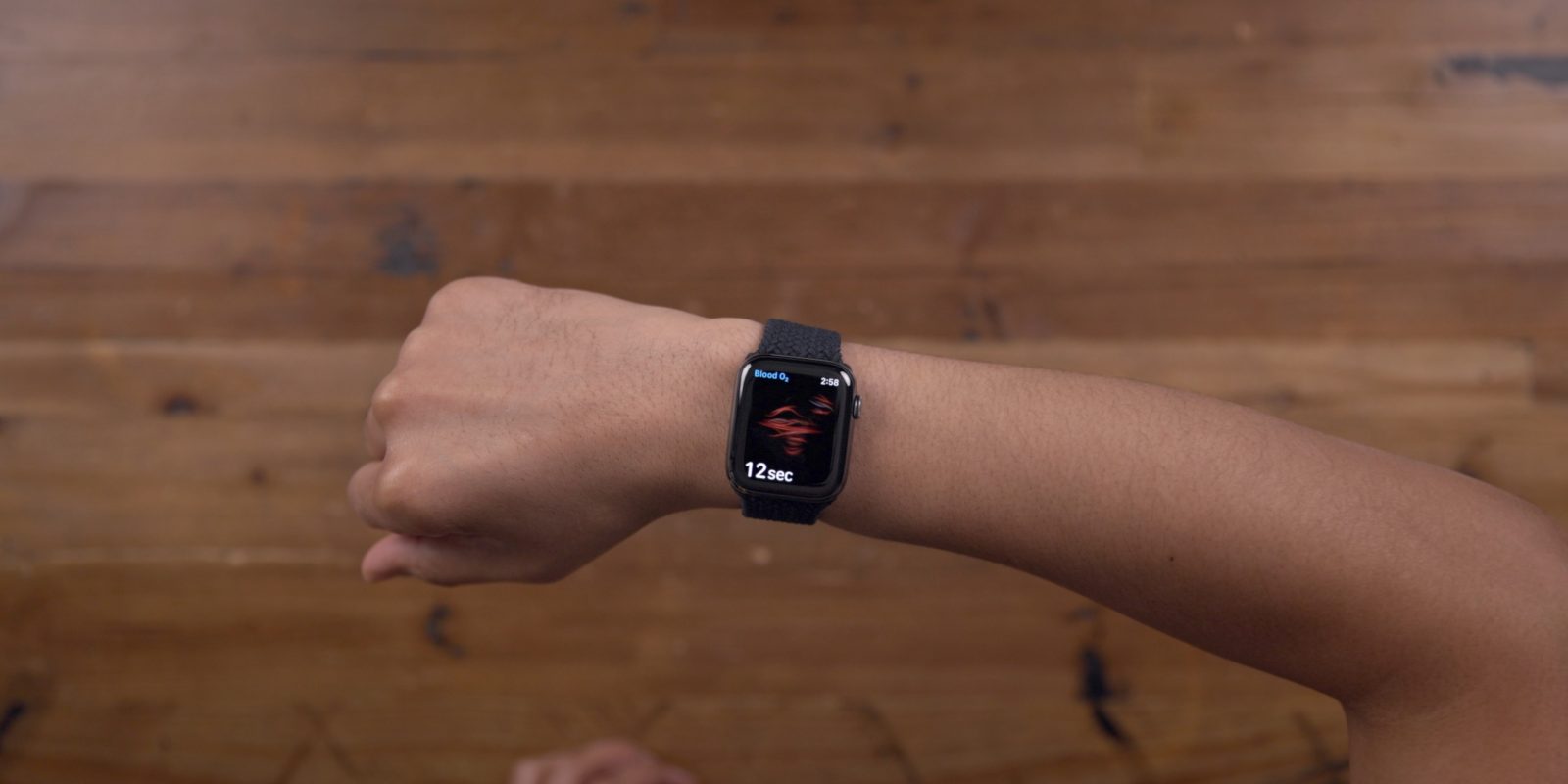
Apple Watch has introduced a host of health benefits over the last six years including monitoring heart health/ECG readings, fall detection, blood oxygen, exercise tracking, and has become more widely used in health studies. However, researchers from Harvard and the University of Michigan have now shared concerns about relying on Apple Watch for studies with the wearable’s algorithms creating “black boxes.”
Reported by The Verge, JP Onnela, an associate professor of biostatistics detailed the potential problems with using Apple Watch for research studies. The particular case he looked at was heart rate variability data collected by Apple Watch, finding it inconsistent, and he thinks the cause could impact other studies too.
The concern hinges on how Apple updates its wearable’s algorithms over time which means “the data from the same time period can change without warning.”
“These algorithms are what we would call black boxes — they’re not transparent. So it’s impossible to know what’s in them,” JP Onnela, associate professor of biostatistics at the Harvard T.H. Chan School of Public Health and developer of the open-source data platform Beiwe, told The Verge.
Devices like Apple Watch are known for exporting information only after being processed through algorithmic filters which can be problematic for “reproducible science.” That’s why Onnela usually sticks to research-grade devices for studies that output raw data, but he was interested to learn more about using Apple Watch in an upcoming study and how notable the potential data problems might be.
So, they checked in on heart rate data his collaborator Hassan Dawood, a research fellow at Brigham and Women’s Hospital, exported from his Apple Watch. Dawood exported his daily heart rate variability data twice: once on September 5th, 2020 and a second time on April 15th, 2021. For the experiment, they looked at data collected over the same stretch of time — from early December 2018 to September 2020.
Onnela was prepared to see some differences in the same data exported at two different times. And their means ended up being similar 52 vs 55, however, the variances showed big differences at 1240 vs 572 and also the same data had a relatively low Pearson linear correlation of 0.67.
Onnela explained more in a blog post:
To be clear, these data cover the same date range, so they should be identical. In fact, their means are very similar, 52 vs. 55 for the first and second export, respectively, but their variances are very different: 1240 vs. 572. To get some further insight into this, I made a scatter plot of the values of one time series against the other. The dashed identity line is where we’d like to see the points fall if they were identical, as we’d hope. Instead, there’s a lot of scatter in the data, and their Pearson linear correlation coefficient is just 0.67. That’s not a very high correlation.
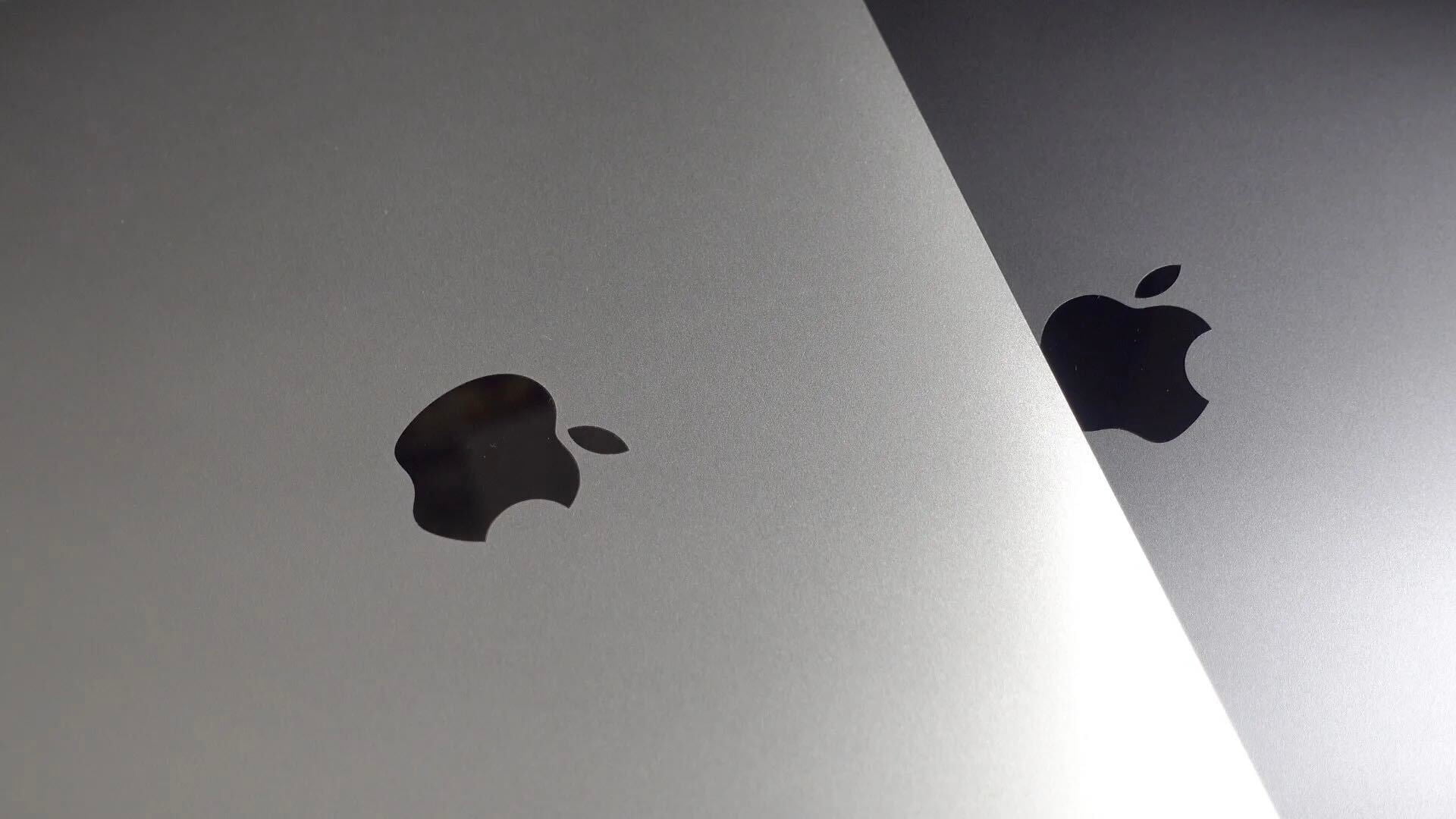
Keep in mind this is just one informal example of Apple Watch data and wasn’t from a research study, but it still was enough to cause concern for Onnela.
Speaking to The Verge, Onnela also used the example of tracking body weight as being impacted by the changing algorithm issues. But it all likely comes down to the type of use:
For someone who has just a casual interest in tracking their health, that may be fine — the differences aren’t going to be major. But in research, consistency matters. “That’s the concern,” he says.
University of Michigan sleep researcher Olivia Walch spoke up about this affirming the use of devices that provide raw data:
“It’s validating, because I get on my little soapbox about the raw data, and it’s nice to have a concrete example where it would really matter,” she says.
Another interesting point around data reliability is different study participants wearing smartwatches that have different software and algorithms.
Constantly changing algorithms makes it almost prohibitively difficult to use commercial wearables for sleep research, Walch says. Sleep studies are already expensive. “Are you going to be able to strap four FitBits on someone, each running a different version of the software, and then compare them? Probably not.”
…
Someone could, for example, run a study using a wearable and come to a conclusion about how people’s sleep patterns changed based on adjustments in their environment. But that conclusion might only be true with that particular version of the wearable’s software. “Maybe you would have a completely different result if you just been using a different model,” Walch says.
However, Walch did say looking for broader trends on a “macro scale” may still be useful with wearables like Apple Watch:
“If you’re caring about stuff on that macro scale, then you can make the call that you’d keep using the device,” Walch says. But if the specific heart rate variability calculated on each day matters for a study, the Apple Watch may be riskier to rely on, she says. “It should give people pause about using certain wearables, if the rug runs the risk of being ripped out underneath their feet.”
Apple didn’t respond to The Verge for a comment on the issue.
FTC: We use income earning auto affiliate links. More.
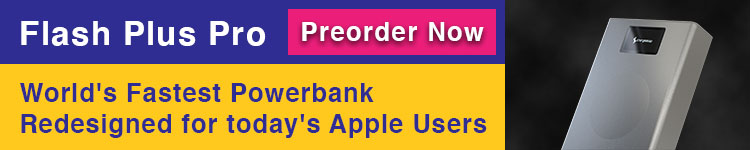
Comments