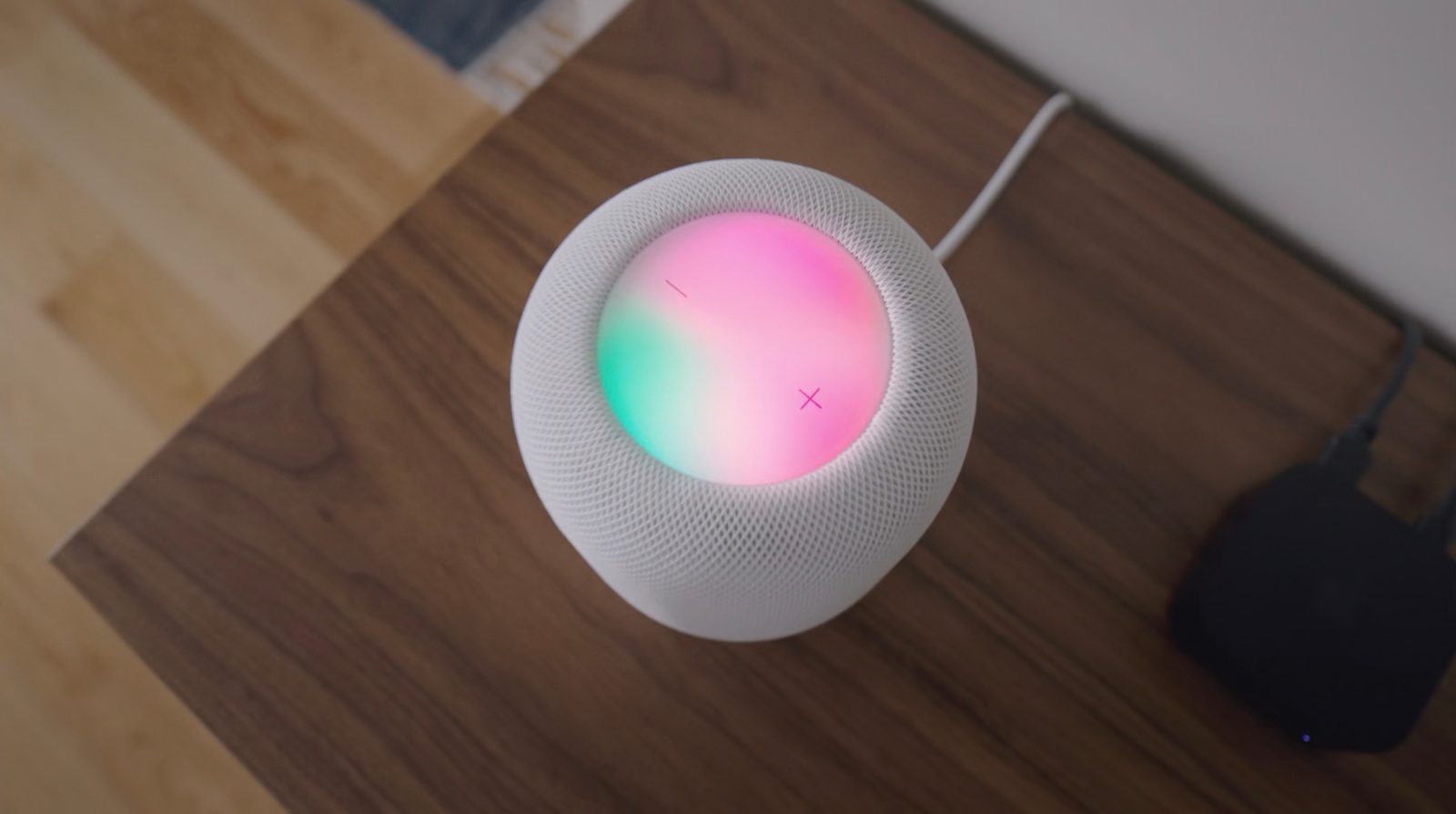
In a new paper published this month, Apple researchers reveal that they have developed new methods for training large language models using both text and visual information. According to Apple’s researchers, this represents a way to obtain state-of-the-art results.
As first spotted by VentureBeat, the idea of the research is to demonstrate “how carefully combining different types of training data and model architectures can lead to state-of-the-art performance on a range of AI benchmarks.”
The paper was published last week and is titled “MM1: Methods, Analysis & Insights from Multimodal LLM Pre-training.” Apple researchers explain in the paper’s abstract:
In this work, we discuss building performant Multimodal Large Language Models (MLLMs). In particular, we study the importance of various architecture components and data choices. Through careful and comprehensive ablations of the image encoder, the vision language connector, and various pre-training data choices, we identified several crucial design lessons.
For example, we demonstrate that for large-scale multimodal pre-training using a careful mix of image-caption, interleaved image-text, and text-only data is crucial for achieving state- of-the-art (SOTA) few-shot results across multiple benchmarks, compared to other published pre-training results.
MM1 is described as a “family of multimodal models” that are state-of-the-art and have “appealing properties such as enhanced in-context learning, and multi-image reasoning, enabling few-shot chain-of-thought prompting.”
The in-context learning capabilities of the MM1 model are particularly impressive:
MM1 can perform in-context predictions thanks to its large-scale multimodal pre-training. This allows MM1 to (a) count objects and follow custom formatting, (b) refer to parts of the images and perform OCR, (c) demonstrate common-sense and word knowledge about everyday objects, and (d) perform basic math functions. Images are from the COCO 2014 validation set.
The researchers conclude that this model family “produces competitive performance on a wide range of benchmarks, while enabling multi-image reasoning and few-shot prompting.”
Read more:
- Apple AI work continues: Editing photos using text commands
- Apple Keyframer generates AI animation from a still image and text prompt
- iOS 18’s new AI features: Everything we know so far
FTC: We use income earning auto affiliate links. More.
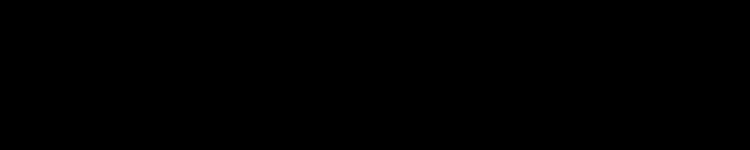
Comments