The Twitter photo-cropping feature, which uses machine learning to decide which part of a photo to show in user feeds, was accused of being racist and sexist last year, when it was found more likely to crop out Black people and women.
The company announced a bug bounty for anyone able to prove biases in its algorithms, and has now named the winners, who variously proved a number of biases …
Background
When you upload a portrait-oriented photo to Twitter, the system automatically makes a crop to display a landscape version in people’s feeds. The full version is shown only when people tap or click on it.
Twitter users started noticing that if a photo showed people with different skin colors, or different sexes, the crop was more likely to show a white man than a Black woman.
The company responded by showing less cropped versions, but also announced an internal investigation and an external competition to prove biases in its algorithms. It has now announced the results of this competition.
Twitter photo-cropping competition results
The Twitter Engineering account tweeted the results, and a link to a video of the presentations (below).
1st place goes to @hiddenmarkov whose submission showcased how applying beauty filters could game the algorithm’s internal scoring model. This shows how algorithmic models amplify real-world biases and societal expectations of beauty.
2nd place goes to @halt_ai who found the saliency algorithm perpetuated marginalization. For example, images of the elderly and disabled were further marginalized by cropping them out of photos and reinforcing spatial gaze biases.
3rd place goes to @RoyaPak who experimented with Twitter’s saliency algorithm using bilingual memes. This entry shows how the algorithm favors cropping Latin scripts over Arabic scripts and what this means in terms of harms to linguistic diversity online.
The most innovative award in the algorithmic bias bounty goes to @OxNaN who explored Emoji-based communication to uncover bias in the algorithm, which favored light skin tone Emojis. This entry shows how well-meaning adjustments to photos can result in shifts to image salience.
The full results confirmed racism, sexism, ageism, ableism, and more. One entrant even found that the algorithm favored lighter skin toned emoji to darker skin toned ones!
Twitter linked to the code for each winning entry on Github.
Twitter’s challenge will now be to fix these biases, which may be a tougher job than proving that they exist.
FTC: We use income earning auto affiliate links. More.
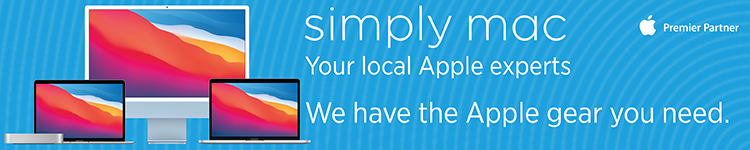
Comments